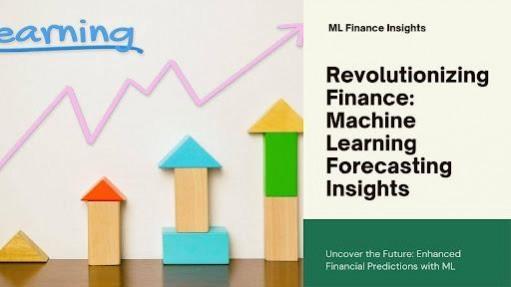
In recent years, machine learning has emerged as a transformative technology in the financial sector, particularly in consumer banking. By leveraging historical data and identifying complex patterns, ML models enable banks to make more accurate predictions and data-driven decisions. According to a survey by the European Banking Authority, 64% of banks in the European Union have already implemented ML in their financial forecasting processes, with an additional 23% planning to do so within the next two years. In this article, you can explore the transformative impact of machine learning (ML) on financial forecasting in consumer banks based on Het Mistry's insights on how ML enhances various banking functions.
Fraud Detection
One of the most significant applications of ML in banking is fraud detection. Traditional methods often fail to detect sophisticated fraudulent activities, but ML models such as Isolation Forest, Autoencoders, and One-Class Support Vector Machines (SVMs) have proven highly effective. These models analyze vast amounts of transaction data, differentiating between normal and suspicious behavior.
For example, JPMorgan Chase's ML model monitors the spending patterns of its 50 million credit card customers in real time, flagging unusual transactions and preventing fraud. Similarly, Danske Bank's real-time ML-based system analyzes over 5 million transactions daily, significantly reducing financial losses due to fraud.
Customer Segmentation and Personalization
Understanding customer needs is crucial for enhancing satisfaction and loyalty. ML techniques, particularly clustering algorithms like K-Means and DBSCAN, enable banks to segment customers based on behavior, spending patterns, and demographics. This segmentation allows for targeted marketing campaigns and personalized product recommendations.
Bank of America, for instance, used ML algorithms to identify 45 distinct customer segments, leading to increased engagement and loyalty. Additionally, recommender systems provide personalized financial product suggestions, improving adoption rates and customer satisfaction. Capital One's ML-based system, which offers customized credit card suggestions, saw a 15% increase in credit card adoption and a 20% boost in customer satisfaction.
Predictive Analytics for Customer Behavior
Anticipating customer behavior is essential for proactive service optimization. ML models, including Logistic Regression and Random Forests, predict customer churn by analyzing behavior patterns. Santander Bank, for example, developed an ML model analyzing over 200 variables to predict account closures.
This approach allowed Santander to implement targeted retention strategies, reducing customer churn by 15%. Moreover, ML models estimate the lifetime value (LTV) of customers, helping banks allocate resources more effectively. HSBC's ML-based LTV prediction model enabled the bank to prioritize high-value customers, optimizing customer acquisition and retention efforts.
Financial Forecasting for Strategic Planning
Accurate financial forecasting is critical for strategic decision-making and risk management. ML models analyze historical financial data and market trends, offering more accurate predictions than traditional methods. JPMorgan Chase's deep learning model, DeepFX, predicts foreign exchange rates with remarkable accuracy, optimizing currency trading strategies and minimizing risk.
Additionally, Machine Learning revolutionizes banking with fraud detection, customer personalization, predictive analytics, and strategic planning. Innovations like Bank of America's stress testing framework highlight ML's transformative impact on financial forecasting.
Interest Rate and Pricing Optimization
Optimizing interest rates and product pricing is complex, requiring consideration of market conditions, competition, and customer risk profiles. ML models enable dynamic adjustments in real-time, using regression analysis and reinforcement learning techniques. Wells Fargo's ML model analyzes customer data and market conditions to determine optimal loan interest rates, improving profitability and customer satisfaction. While Citibank's LSTM-based model forecasts interest rate movements, optimizing its bond portfolio and reducing exposure to interest rate risk.
In all, ML is revolutionizing financial forecasting in consumer banking, enhancing fraud detection, personalizing customer experiences, predicting behavior, aiding strategic planning, and optimizing pricing. As ML technologies advance, their application in financial forecasting will continue to transform consumer banking, ensuring banks remain competitive in a data-driven industry.