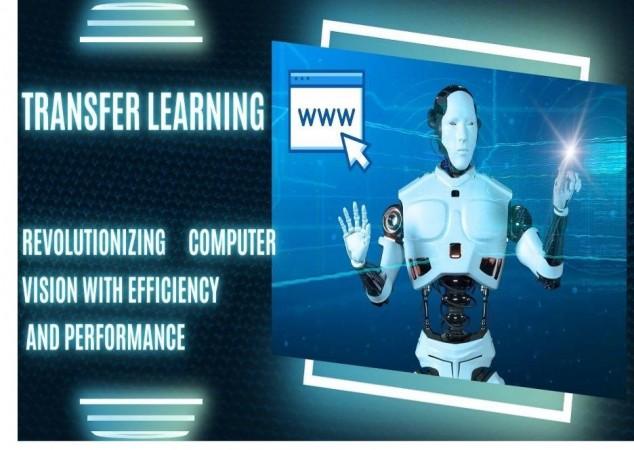
The landscape of computer vision is evolving rapidly, driven by the increasing complexity of tasks such as image classification, object detection, and facial recognition. However, traditional methods that rely on vast datasets and significant computational resources are becoming inefficient. In response to these challenges, Rathish Mohan, a visionary in artificial intelligence, introduces the concept of transfer learning, which significantly enhances efficiency and performance in computer vision tasks. He has extensively researched the potential of transfer learning to overcome existing limitations, making it an essential tool for the future of AI-driven technologies.
Overcoming Data Challenges in Computer Vision
One of the most significant barriers to effective computer vision training is the need for enormous amounts of labeled data. Traditional deep learning models require millions of labeled images to achieve high accuracy, which is both time-consuming and expensive. Transfer learning provides an innovative solution by using pre-trained models that have already learned basic features from large datasets. These models can be fine-tuned for specific tasks with much smaller datasets, reducing both the time and cost of data collection and labeling.
Reducing Computational Resource Requirements
Training deep learning models from scratch demands substantial computational power. Transfer learning mitigates this by using pre-trained models, making the training process faster and more efficient. Fine-tuning these models for specific tasks reduces resource requirements, allowing smaller organizations to leverage advanced AI technologies without needing expensive hardware..
Fine-tuning for Task-Specific Applications
The success of transfer learning lies in its ability to adapt pre-trained models for various computer vision tasks. By fine-tuning models such as ResNet or EfficientNet, which have been trained on large datasets, researchers can apply them to specific tasks like medical imaging or facial recognition with great success. This flexibility allows models to excel in a wide range of domains, from medical diagnostics to autonomous driving, without the need for vast amounts of task-specific data.
Feature Extraction for Efficient Model Performance
One of the key strengths of transfer learning is its ability to extract useful features from pre-trained models. These models, which have already learned general patterns from large datasets, can identify relevant features even in new, domain-specific tasks. For example, a model trained on general images can be fine-tuned to recognize specific textures or patterns in medical images. This feature extraction process allows models to achieve high performance without the need for extensive retraining.
Advantages of Transfer Learning in Diverse Domains
Transfer learning not only improves efficiency but also enhances model performance with limited data. In fields like healthcare and specialized industries, where large datasets are scarce, it enables AI models to achieve impressive results, such as state-of-the-art performance in medical imaging tasks like tumor detection and disease classification.
Future Directions for Transfer Learning
As the field of artificial intelligence continues to advance, transfer learning is poised to play an even more significant role in computer vision. Researchers are exploring new techniques such as meta-learning and multi-task learning, which aim to make models more adaptable and generalizable across various tasks. Additionally, the integration of unsupervised learning methods and neural architecture search promises to further enhance the capabilities of transfer learning models, pushing the boundaries of what is possible in AI-driven technologies.
In conclusion, Rathish Mohan emphasizes that transfer learning represents a major leap forward in computer vision, offering a more efficient, flexible, and powerful approach to solving complex tasks. By reducing the need for extensive data and computational resources, transfer learning not only democratizes access to advanced AI technologies but also improves the performance of models across a wide range of applications. As the technology continues to evolve, it will undoubtedly become an essential tool for future innovations in AI and computer vision.