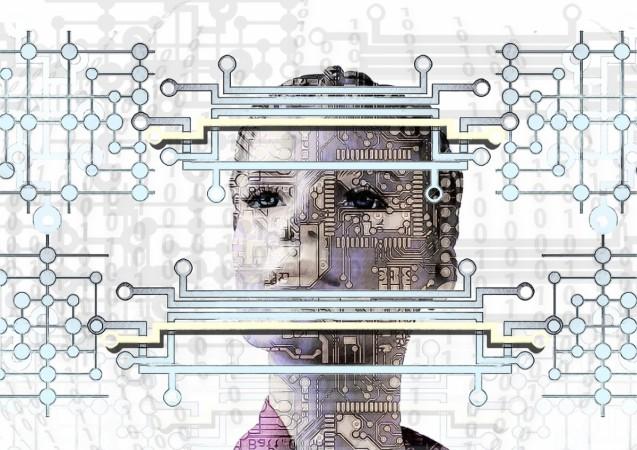
The human brain is far more complicated than most computers. Its vast network of neurons are able to process information fast and use little power. Computer scientists are not even close to achieving brain level functionality, but they are getting close.
A recently published study explores the building of a physical neural network that works exactly like a brain. This network even has circuitry that closely resembles neurons. When tests were run on this network using an artificial intelligence program, it was found that not only did the new network function on par with traditional systems, it actually performed the tasks using much less power.
According to a report on the study by the MIT Technology Review, the new integrated neural net system was able to complete similar tasks with 100 times less energy. "Chips built this way might turbocharge machine learning in coming years," notes the report.
Neuron-based circuits can accomplish a lot more computing using much less energy than conventional computers, say the researchers. The report likens the process of AI communicating with a computer as a telephone connected to a tin can with a string, slowing down the entire process. With a neural net system, both the AI and its processors speak "the same language" and they are able to communicate without any drops in accuracy.
Mimicking the human brain
The chip made by IBM mimics the way virtual neural nets are written in software, but the chip is made of silicon and connect to each other like synapses in the human brain. To teach the network to learn anything at all, the strength of these connections have to be tuned accordingly, says the report.
Just like a human brain, synaptic connections get stronger and weaker over time as the brain learns new things. While this process can be easily mimicked in software, this is the first time it has been made possible through hardware.
Researchers have a taken inspiration from the neurosciences by making use of two types of synapses – short and long-term. Short-term synapses will be used for computation and long term synapses for memory.
Using this method, the AI was handed two simple tasks that deal with image recognition – handwriting, and colour classifications. When compared to the same tasks performed by software-based deep learning algorithms, the physical neural network performed the tasks with the same amount of accuracy, but consumed only one percent of the power needed, says the report.
"A factor of 100 in energy efficiency and in training speed for fully connected layers certainly seems worth further effort," says Michael Schneider, a researcher at that National Institute of Standards and Technology.
The paper was first published in the journal Nature.
.