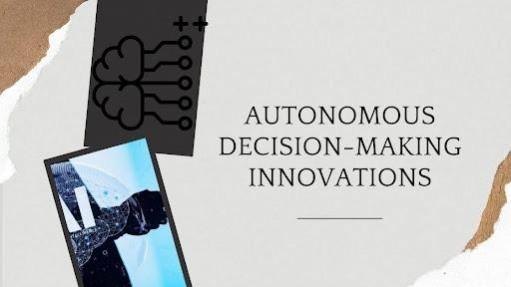
Reinforcement learning (RL) is reshaping the future of artificial intelligence, allowing machines to learn, adapt, and make decisions in dynamic environments. From mastering complex games to revolutionizing industries like robotics, healthcare, and autonomous driving, RL's potential is vast and transformative. As the technology evolves, it presents exciting opportunities and unique challenges, particularly in scaling and ethical deployment. In this exploration, you'll discover how RL drives innovation and shapes tomorrow's AI landscape, as highlighted by Pradeep Sambamurthy's comprehensive research.
The Essence of Reinforcement Learning
Reinforcement learning (RL), inspired by behavioral psychology, enables agents to learn through trial and error, guided by rewards and penalties. Unlike traditional AI paradigms like supervised learning, which relies on labeled data, RL interacts with environments to optimize cumulative rewards. It has been applied in diverse fields, from gaming AI to robotics and healthcare. By incorporating deep learning and neural networks, RL systems can process complex, high-dimensional data and make optimized decisions in real time, making RL invaluable for applications requiring adaptive autonomous behavior in dynamic environments.
Key Innovations in RL
One of the most notable advancements in reinforcement learning (RL) is DeepMind's AlphaGo, a system that defeated the world champion in Go, a game renowned for its complexity. This milestone demonstrated RL's capacity to master long-term planning and strategic thinking tasks. Beyond gaming, RL is transforming robotics, with algorithms enabling machines to perform intricate tasks like assembling parts with precision.
Robotic arms powered by RL have reduced task completion times by 30%, improving manufacturing efficiency. RL is also making strides in autonomous driving, where some companies use RL to train vehicles in simulated environments. These simulations allow autonomous cars to navigate complex traffic scenarios, enhancing safety and efficiency through real-time decision-making based on extensive driving data.
RL in Healthcare: Personalized Treatment and Beyond
One of the most exciting applications of RL is in the healthcare industry. Researchers are now exploring RL algorithms to develop personalized treatment plans for patients with chronic diseases. For instance, RL systems have been utilized to optimize radiation therapy for cancer patients, minimizing side effects while enhancing treatment efficacy.
In a study highlighted by Pradeep Sambamurthy, an RL algorithm outperformed human clinicians in managing sepsis treatment. The system analyzed data from thousands of patients, determining optimal treatment strategies that reduced mortality rates by up to 8.5%. This achievement underscores the potential of RL to revolutionize patient care by providing more accurate and timely interventions.
Tackling Real-World Challenges
Although RL excels in controlled environments like games, real-world applications face challenges, including the need for massive data and computational resources. Training models like OpenAI's GPT-3 required extensive GPU time and significant financial investment in computing power.
Researchers are addressing RL's challenges by developing hybrid approaches that integrate model-based methods and transfer learning. These approaches enable systems to generalize knowledge across tasks. Additionally, distributed RL accelerates training by parallelizing learning, enhancing scalability for real-world applications.
Future Prospects and Ethical Considerations
As reinforcement learning (RL) advances, it raises ethical and societal concerns, especially in critical areas like autonomous vehicles and healthcare. Ensuring RL systems are safe, reliable, and unbiased is crucial for responsible AI development. Multi-agent RL, where systems cooperate or compete, introduces new opportunities in swarm robotics, smart cities, and decentralized finance. These emerging applications require new protocols for communication and coordination, pushing RL research into uncharted territories and highlighting the need for robust ethical frameworks to guide development.
To sum up, reinforcement learning (RL) is revolutionizing AI by tackling complex, real-world challenges through interaction, adaptation, and optimized decision-making. Its broad applications, from improving manufacturing efficiency to enhancing healthcare outcomes, demonstrate RL's transformative potential. The future of RL is bright, with advancements in multi-agent learning, meta-learning, and safe exploration set to push AI capabilities even further. However, the challenge lies in scaling these innovations while addressing the ethical concerns they raise, ensuring responsible and impactful AI development in the coming decade.