A new machine learning (ML) platform can identify patients with and helps predict their disease severity and survival finds a new study. The non-invasive method adds to recent advances in technologies that analyse circulating tumour DNA (ctDNA) and could help spot colorectal cancers in at-risk patients at earlier stages.
![Six members of a family residing in the Vadodara district of Gujarat, India, were diagnosed with an extremely rare form of cancer, called Familial Adenomatous Polyposis. [Representational image] doctor](https://data1.ibtimes.co.in/en/full/686204/doctor.jpg?h=450&l=50&t=40)
Like many other malignancies, colorectal cancers are most treatable if they are detected before they have metastasized to other tissues. Colonoscopies are the 'gold standard' for diagnosis, but they are uncomfortable and invasive and can lead to complications, which leaves patients less willing to undergo screening.
For the study, published in the journal Science Translational Medicine, lead researcher Huiyan Luo from University Cancer Center in China and colleagues leveraged machine learning techniques to develop a less invasive diagnostic method that can detect colorectal cancer in at-risk patients. Their technology works by screening for methylation markers, which are DNA modifications that are frequently found in tumors.
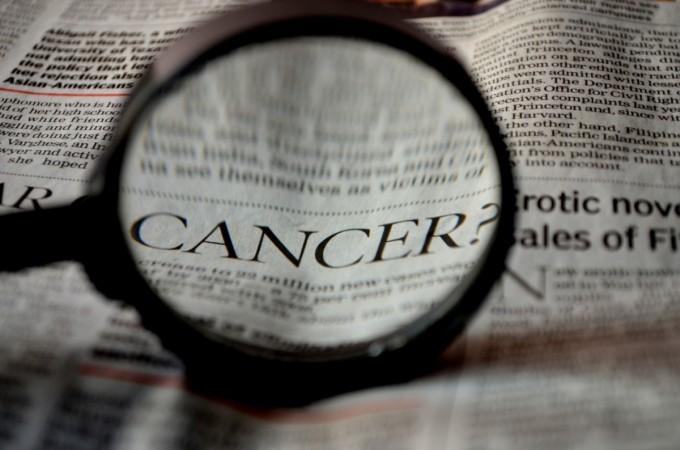
The researchers first created a diagnostic model based on nine methylation markers associated with colorectal cancer, which they identified by studying plasma samples from 801 patients with colorectal cancer as well as 1,021 controls. This model accurately distinguished patients from healthy individuals with a sensitivity and specificity of 87.5 per cent and 89.9 per cent, respectively, and outperformed a clinically available blood test named CEA, the study said.
New model helped predict patients' risk of death
Furthermore, a modified prognostic model helped predict the patients' risk of death over a follow-up period of 26.6 months on average, especially when combined with established clinical characteristics such as tumor location.
One methylation marker was particularly useful, as screening for it alone spotted cases of colorectal cancer and precancerous lesions in a prospective study of 1,493 at-risk individuals, according to the researchers. The researchers concluded that studies with longer follow-up periods will be needed to further assess their model's reliability for clinicians and patients.