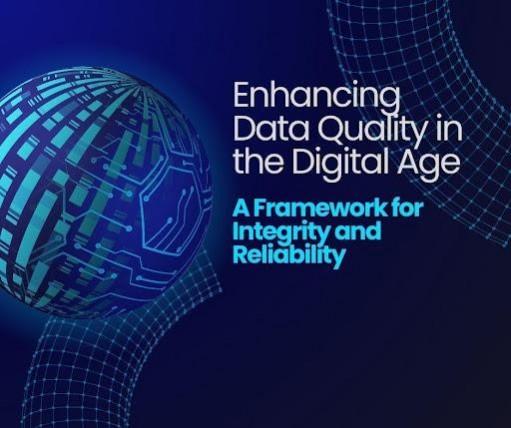
In today's data-driven business landscape, ensuring the integrity, accuracy, and reliability of data has become more crucial than ever. Srikanth Gangarapu and co-authors Vishnu Vardhan Reddy Chilukoori, Abhishek Vajpayee, and Rathish Mohan present an insightful framework that tackles these challenges, providing organizations with the tools to maintain data excellence. This article explores key innovations in data quality assurance, offering a comprehensive roadmap for businesses to navigate the complexities of data warehousing.
Addressing Common Data Quality Issues
Modern data warehouses face numerous quality issues like duplicates, inconsistencies, missing values, and data drift, often caused by complex data integration and diverse sources. The authors propose a proactive data management framework, addressing these challenges with tools like matching algorithms for duplicates and standardization for inconsistencies. By systematically resolving these issues, organizations can improve decision-making and gain more accurate insights from their data.
Best Practices for Ensuring Data Integrity
For long-term success in data quality management, essential practices such as data validation, reconciliation, and preventive measures are key. Automated validation rules embedded throughout the data pipeline ensure data conforms to required formats, preventing errors from the outset. Reconciliation techniques, including cross-system comparisons and historical data checks, help identify and correct inconsistencies, ensuring ongoing accuracy. Preventive measures like data profiling and metadata management further reduce errors, enhancing operational efficiency. Together, these practices minimize inaccuracies, enabling businesses to make more reliable, data-driven decisions and maintain data integrity.
Leveraging Technology for Advanced Data Monitoring
Automated monitoring systems have revolutionized data quality management by enabling real-time tracking and immediate detection of discrepancies, ensuring continuous oversight of data accuracy. These systems allow organizations to swiftly address issues as they emerge, preventing small errors from escalating into larger problems. The integration of machine learning further elevates this process by identifying subtle anomalies that traditional methods might overlook, offering proactive and intelligent solutions to complex data challenges. This dynamic, AI-driven approach not only helps maintain reliable data but also ensures compliance with industry standards, enhancing overall operational efficiency and decision-making.
Data Governance and Regulatory Compliance
As regulatory requirements become more stringent, the need for robust data governance structures is critical. The framework underscores the role of governance and stewardship in establishing and enforcing data quality standards. By adopting comprehensive governance policies, organizations can ensure regulatory compliance while fostering a culture of accountability and excellence. The authors highlight the intersection of data quality assurance and compliance, showing how governance supports adherence to complex regulations. By leveraging measures such as lineage tracking, encryption, and privacy safeguards, businesses can protect sensitive data, meet legal obligations, and maintain customer trust.
The Future of Data Quality Management
The future of data quality assurance lies in the integration of artificial intelligence, real-time monitoring, and adaptive governance models, which are poised to transform how organizations manage their data. Real-time monitoring offers continuous oversight, minimizing the risk of outdated information influencing decision-making. AI-driven governance models provide flexible approaches to managing data quality in complex, distributed environments. By embracing these advancements, organizations can stay agile, maintain a competitive edge, and unlock the full potential of their data assets in an evolving landscape.
In conclusion, the data quality assurance framework presented by Srikanth Gangarapu and his co-authors offers a holistic approach to managing data integrity, accuracy, and reliability in modern data warehousing environments. By addressing common data quality challenges, implementing best practices, and leveraging advanced technologies, organizations can ensure that their data remains a valuable asset for driving innovation and informed decision-making. As businesses continue to rely on data to navigate the complexities of the digital age, the importance of maintaining high-quality data cannot be overstated.