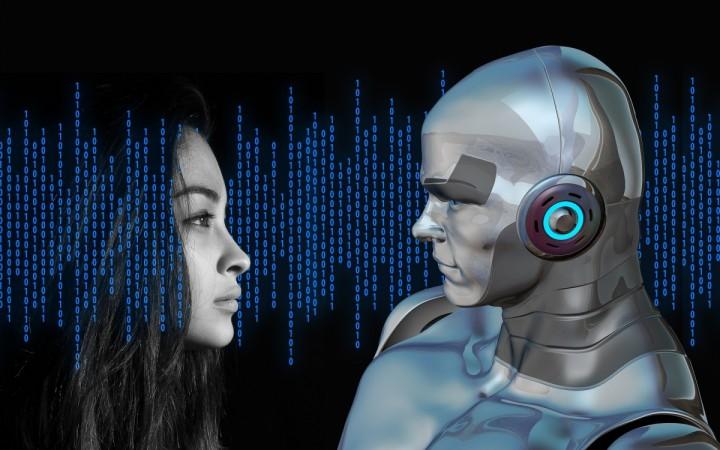
A deep neural network has been taught how to process sound and replicate human performance with audio-related tasks. The way this artificial intelligence (AI) program works is similar to the way human brains work with regards to sound.
The model consists of several layers of information processing and can be trained using large volumes of data to perform specific tasks. This experiment has also shed light on the way the human brain works while processing sound, notes a report by MeedicalXpress. The research was carried out by a team from MIT.
"What these models give us, for the first time, is machine systems that can perform sensory tasks that matter to humans and that do so at human levels," says Josh McDermott from the Department of Brain and Cognitive Sciences at MIT and senior author of this study.
"Historically, this type of sensory processing has been difficult to understand, in part because we haven't really had a very clear theoretical foundation and a good way to develop models of what might be going on."
This study has opened up a new way to approach the auditory cortex, notes the report. In fact, there is now evidence to prove that the human brain makes use of a hierarchical organization in information processing, similar to the way the brain processes vision and images. What that means is that sensory information is put through several stages of processing. When a person sees a signboard, for example, this information is put through a stages where basic information is understood first and advanced features like meaning of the words is "extracted" in later stages.
For this experiment, the MIT team trained a neural network to carry out two sound processing tasks – recognise speech and music. The speech task had researchers feed thousands of two-second recordings of a person talking. The AI had to understand and identify the word in the middle of the clip. The music task was similar, but the program needed to find out the genre of music played in the two-second clips. After thousands of attempts and learning, with background noises as well added to give it a more real-world feel, the AI was able to perform the tasks just as well as human subjects, say researchers.
"The idea is over time the model gets better and better at the task," says Alexander Kell, one of the lead authors of the study. "The hope is that it's learning something general, so if you present a new sound that the model has never heard before, it will do well, and in practice that is often the case."
Neural networks were first developed in the 1980s, notes the report, and modeling the human brain is one of the things that scientists have always wanted to accomplish. Computers of that time, however, were just not powerful enough to carry out tasks like object and speech recognition. However, over the last five years, computing has grown to an extent where making machines perform real-world tasks has become sort of the norm so scientists have now come back to the possibility of a neural network to model the human brain.
"That's been an exciting opportunity for neuroscience, in that we can actually create systems that can do some of the things people can do, and we can then interrogate the models and compare them to the brain," says Kell.