The ability of artificial intelligence (AI) and machine learning (ML) in furthering medical research has become indisputable. Adding credence to their application, a new ML algorithm has helped researchers identify over 160 new genes that are responsible for the development of numerous cancers.
Developed by scientists from the Max Planck Institute for Molecular Genetics (MPIMG) and Institute of Computational Biology (ICB), the algorithm helped identify 165 unknown cancer genes. The new genes are said to closely interact with previously known cancer-causing genes and found to be vital in the survival of cancer cells in cell culture experiments.
Talking about the potential use of the algorithm for other diseases, Annalisa Marsico, group leader of the research, said in a statement, "It could be useful to apply our algorithm for similarly complex diseases for which multifaceted data are collected and where genes play an important role. An example might be complex metabolic diseases such as diabetes."
Identifying New Cancer Genes
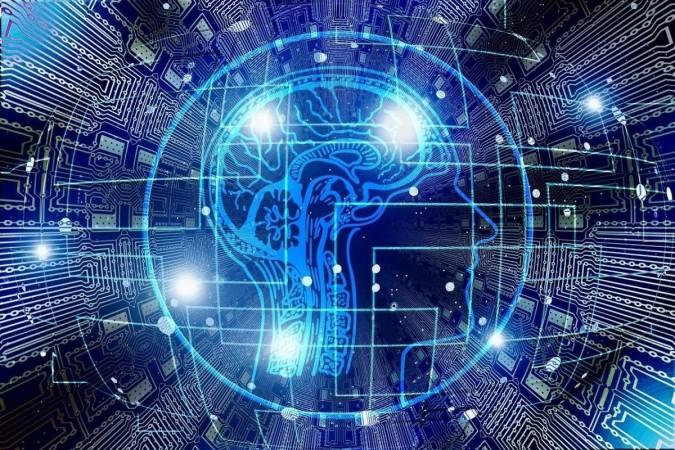
When an individual is afflicted with cancer, the growth of cancerous cells is aggressive. Their rapid multiplication can invade other tissues and damage organs, thereby, impeding their functions. Generally, the unrestrained growth of these cells is triggered by the accrual of DNA changes or mutations in cancer genes. However, in some cancers, a limited number of mutated genes are involved. This means that other factors may contribute to the expeditious proliferation of such cancer cells.
Known as Explainable Multi-Omics Graph Integration (EMOGI), the algorithm is based on graph convolutional networks (GCN). It was able to identify 165 unknown cancer genes. The alterations in the sequences of these genes are not definite. However, an ongoing dysregulation—abnormality of impairment in the regulatory process—in these genes can lead to the development of cancer.
The use of deep learning algorithms in recent years has led to the expansion of the list of suspected cancer genes to around 700 to 1,000. Helping increase these numbers, the new algorithm analyzed thousands of distinct network maps from 16 different cancer types—each of which contained between 12,000 and 19,000 data points.
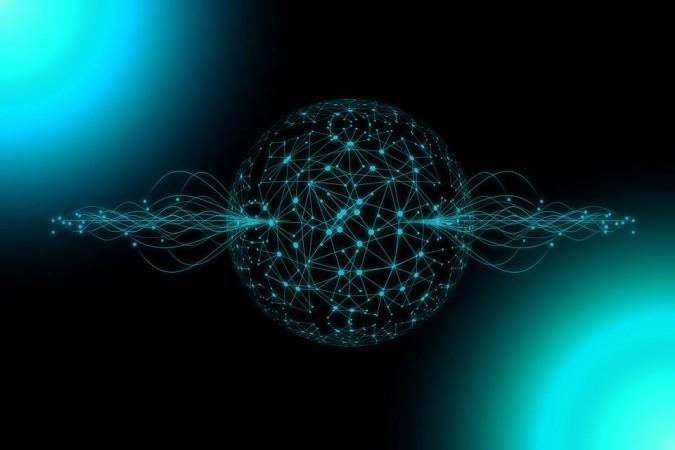
EMOGI consolidated thousands of datasets acquired from patient samples. Using this, it illustrated the connections within a cell's machinery that transformed a gene into a cancer gene. Important information about DNA methylations is contained within the aggregated data. DNA Methylation is the process through which methyl groups get attached or added to the DNA molecules. Utilizing this data, the algorithm identified molecular principles and patterns that give rise to cancer and its development.
A Blended Approach
Roman Schulte-Sasse, the lead author of the study, stated that most cancer research has centered on pathogenic changes within the genetic sequence (blueprint) of cells. "At the same time, it has become apparent in recent years that epigenetic perturbations or dysregulated gene activity can lead to cancer as well," explained Schulte-Sasse. Therefore, the authors employed a blended approach in their research.
They combined sequence data the reflected defects within the genetic sequence with information that was representative of intra-cellular activity. At the very beginning, the researchers confirmed that mutations are the primary promotes of cancer. Next, they located genes that had less direct involvement in the framework of the real cancer-promoting genes.
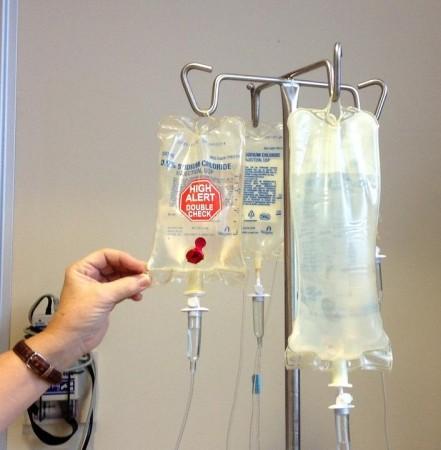
Schulte-Sasse pointed out that while such genes were "promising drug targets", they can be found only through the use of complex algorithms as these genes operate in the background.
New Targets for Personalized Treatment
Currently, therapies such as chemotherapy and immunotherapy are the standard treatment for cancers. However, such traditional therapies are general in nature and have a vital drawback—the absence of individualization. According to the team, this algorithm may help in the formulation of individual treatment.
"The goal is to select the best therapy for each patient – that is, the most effective treatment with the fewest side effects. Additionally, we would be able to identify cancers already at early stages, based on their molecular characteristics," said Marsico.
She also highlighted the importance of identifying the several mechanisms that induce cancer. "Only if we know the causes of the disease will we be able to counteract or correct them effectively," she concluded.